一、背景
随着数据仓库的数据量的增长,数据血缘( Data Lineage or Data Provence ) 对于数据分析来说日益重要, 通过数据血缘可以追溯表-表,表-任务,任务-任务的上下游关系, 用来支撑问题数据溯源,孤岛数据下线的需求。
目前已经基于 ANTLR 语法解析支持了 SQL 任务的血缘解析, 而 Spark App 任务的血缘仍然是通过人工配置方式进行。 我们希望能够将 Spark App 任务的解析做个补充,完善血缘逻辑。
目前线上的 Spark App 任务支持 Spark 2.3、 Spark 3.1 两个版本, 并且支持 python2/3、 java、scala 类型, 运行平台各自支持 yarn 和 k8s, 血缘的收集机制需要考虑适配所有上述所有任务。
二、思路
可以想到的 Spark App 任务的解析思路有以下三类:
- 基于代码解析: 通过解析 Spark App 的逻辑去达到血缘解析的目的, 类似的产品有 SPROV[1]
- 基于动态监听: 通过修改代码达到运行时收集血缘的目的的 Titian [2] 和 Pebble [3] , 或者通过插件方式在运行时收集血缘的 Spline [4] 和 Apache Atlas [5]
- 基于日志解析: 通过分析例如 Spark App 的 event log 信息,然后解析出任务的血缘
因为 Spark App 的写法多样, 基于代码的解析需要考虑java、python、 scala, 显得过于复杂, 我们首先考虑了基于日志的分析。 通过分析 spark3 和 spark2 的任务的历史 event log 发现, spark2 的 event log 没有完整的 hive表 相关的元信息, 而 spark3 则在各种读取算子例如 FileSourceScanExec 和 HiveTableScan 的基础上打印出了 hive 表元信息, 所以基于 event log 方式不能完美支持 spark2 。
所以基于此我们最终打算采用基于动态监听的方式,并且调研了 spline, 进行了可用性分析。 下面介绍下 spline 的使用和设计原理。
三、spline
3.1 spline 原理
spline (Spark Lineage)是一个免费基于 Apache 2.0 协议开源的 Spark 血缘收集系统。该系统主要分为三部分: spline agent、 spline server 和 spline ui。
这里主要介绍 spline agent 的原理, 因为这是负责血缘解析的部分, 至于 spline server 和 ui 就负责血缘的收集和展示,可以用内部的系统替换。
总架构图如下图所示:
3.1.1 初始化
spline支持两种初始化方式,codeless 和 programmatic。本质上都是注册一个 QueryExecutionListener
, 负责监听 SparkListenerSQLExecutionEnd
消息。
codeless 初始化
codeless init 就是通过配置化方式嵌入用户的 Spark APP 程序, 而不需要修改代码。通过注册 QueryExecutionListener 监听器,可以接收并且处理 Spark 的消息。 启动配置例如:
spark-submit --jars /path/to/lineage/spark-3.1-spline-agent-bundle_2.12-1.0.0-SNAPSHOT.jar --files /path/to/lineage/spline.properties --num-executors 2 --executor-memory 1G --driver-memory 1G --name test_lineage --deploy-mode cluster --conf spark.spline.mode=BEST_EFFORT --conf spark.spline.lineageDispatcher.http.producer.url=http://172.18.221.156:8080/producer --conf "spark.sql.queryExecutionListeners=za.co.absa.spline.harvester.listener.SplineQueryExecutionListener" test.py
通过 --jars 执行 spline agent jar 包地址, 也可以默认放到 spark 部署的 jars 目录下
通过 --files 指定 spline properties 文件, 也可以直接通过 --conf 指定配置项,配置项需要额外加上 spark. 前缀
通过 --conf "spark.sql.queryExecutionListeners=za.co.absa.spline.harvester.listener.SplineQueryExecutionListener" 可以注册监听器
programmatic 初始化
programmatic init 需要在代码中显示的开启血缘解析, 例如
- scala demo
// given a Spark session ...
val sparkSession: SparkSession = ???
// ... enable data lineage tracking with Spline
import za.co.absa.spline.harvester.SparkLineageInitializer._
sparkSession.enableLineageTracking()
- java demo
import za.co.absa.spline.harvester.SparkLineageInitializer;
// ...
SparkLineageInitializer.enableLineageTracking(session);
- python demo
from pyspark.sql import SparkSession
from pyspark.sql import functions as F
spark = SparkSession.builder \
.appName("spline_app")\
.config("spark.jars", "dbfs:/path_where_the_jar_is_uploaded")\
.getOrCreate()
sc = spark.sparkContext
sc.setSystemProperty("spline.mode", "REQUIRED")
jvm = sc._jvm
sc._jvm.za.co.absa.spline.harvester.SparkLineageInitializer.enableLineageTracking(spark._jsparkSession)
3.1.2 血缘解析
血缘解析逻辑在 SplineAgent.handle()
方法。通过调用 LineageHarvester.harvest()
获取最终的血缘, 并交给 LineageDispatcher
输出结果。
通过 SparkListenerSQLExecutionEnd
消息可以获取到消息中的 QueryExecution
, 血缘解析基于 QueryExecution
中的 analyzed logical plan 和 executedPlan 进行, LineageHarvester.harvest()
逻辑处理如下:
tryExtractWriteCommand (logicalPlan)
负责解析出 logicalPlan 中的写操作。 写操作的解析依托于插件方式。通过获取
PluginRegistry
中WriteNodeProcessing
类型的插件, 获取 logicalPlan 中的写操作,通过对具体的 Command 的解析,可以获取到例如 hive 表的 表名信息。最后信息会封装为WriteCommand
数据结构。例如
DataSourceV2Plugin.writeNodeProcessor()
会负责V2WriteCommand
、CreateTableAsSelect
、ReplaceTableAsSelect
这几个命令的解析。解析插件可以自己扩展,丰富 spline 解析的数据源, 插件需要继承
za.co.absa.spline.harvester.plugin.Plugin
, spline agent 会在启动的时候自动加载 classpath 中的所有插件。解析到 writeCommand 以后会基于 writeCommand 中的 query 字段解析读操作。读操作基于 query 这部分 logicalPlan 进行递归解析
最后解析完成可以得到 plan 和 event 两个 json 信息,plan 为血缘关系, event 为额外的辅助信息。
例如:
[
"plan",
{
"id": "acd5157c-ddc5-5ef0-b1bc-06bb8dcda841",
"name": "team evaluation ranks",
"operations": {
"write": {
"outputSource": "hdfs:///user/hive/warehouse/dm_ai.db/dws_kdt_comment_ranks_info",
"append": false,
"id": "op-0",
"name": "CreateDataSourceTableAsSelectCommand",
"childIds": [
"op-1"
],
"params": {
"table": {
"identifier": {
"table": "dws_kdt_comment_ranks_info",
"database": "dm_ai"
},
"storage": "Storage()"
}
},
"extra": {
"destinationType": "orc"
}
},
"reads": [
{
"inputSources": [
"hdfs://yz-cluster-qa/user/hive/warehouse/dm_ai.db/dws_kdt_comment_rank_base"
],
"id": "op-6",
"name": "LogicalRelation",
"output": [
"attr-0",
"attr-1",
"attr-2",
"attr-3",
"attr-4",
"attr-5",
"attr-6",
"attr-7",
"attr-8",
"attr-9",
"attr-10",
"attr-11",
"attr-12"
],
"params": {
"table": {
"identifier": {
"table": "dws_kdt_comment_rank_base",
"database": "dm_ai"
},
"storage": "Storage(Location: hdfs://yz-cluster-qa/user/hive/warehouse/dm_ai.db/dws_kdt_comment_rank_base, Serde Library: org.apache.hadoop.hive.ql.io.orc.OrcSerde, InputFormat: org.apache.hadoop.hive.ql.io.orc.OrcInputFormat, OutputFormat: org.apache.hadoop.hive.ql.io.orc.OrcOutputFormat, Storage Properties: [serialization.format=1])"
}
},
"extra": {
"sourceType": "hive"
}
}
],
"other": [
{
"id": "op-5",
"name": "SubqueryAlias",
"childIds": [
"op-6"
],
"output": [
"attr-0",
"attr-1",
"attr-2",
"attr-3",
"attr-4",
"attr-5",
"attr-6",
"attr-7",
"attr-8",
"attr-9",
"attr-10",
"attr-11",
"attr-12"
],
"params": {
"identifier": "spark_catalog.dm_ai.dws_kdt_comment_rank_base"
}
},
{
"id": "op-4",
"name": "Filter",
"childIds": [
"op-5"
],
"output": [
"attr-0",
"attr-1",
"attr-2",
"attr-3",
"attr-4",
"attr-5",
"attr-6",
"attr-7",
"attr-8",
"attr-9",
"attr-10",
"attr-11",
"attr-12"
],
"params": {
"condition": {
"__exprId": "expr-0"
}
}
},
{
"id": "op-3",
"name": "Project",
"childIds": [
"op-4"
],
"output": [
"attr-0",
"attr-1",
"attr-2",
"attr-3",
"attr-4",
"attr-5",
"attr-6",
"attr-7",
"attr-8",
"attr-9",
"attr-10",
"attr-11",
"attr-12"
],
"params": {
"projectList": [
{
"__attrId": "attr-0"
},
{
"__attrId": "attr-1"
},
{
"__attrId": "attr-2"
},
{
"__attrId": "attr-3"
},
{
"__attrId": "attr-4"
},
{
"__attrId": "attr-5"
},
{
"__attrId": "attr-6"
},
{
"__attrId": "attr-7"
},
{
"__attrId": "attr-8"
},
{
"__attrId": "attr-9"
},
{
"__attrId": "attr-10"
},
{
"__attrId": "attr-11"
},
{
"__attrId": "attr-12"
}
]
}
},
{
"id": "op-2",
"name": "Project",
"childIds": [
"op-3"
],
"output": [
"attr-0",
"attr-1",
"attr-2",
"attr-3",
"attr-4",
"attr-5",
"attr-6",
"attr-7",
"attr-8",
"attr-9",
"attr-10",
"attr-11",
"attr-12",
"attr-13"
],
"params": {
"projectList": [
{
"__attrId": "attr-0"
},
{
"__attrId": "attr-1"
},
{
"__attrId": "attr-2"
},
{
"__attrId": "attr-3"
},
{
"__attrId": "attr-4"
},
{
"__attrId": "attr-5"
},
{
"__attrId": "attr-6"
},
{
"__attrId": "attr-7"
},
{
"__attrId": "attr-8"
},
{
"__attrId": "attr-9"
},
{
"__attrId": "attr-10"
},
{
"__attrId": "attr-11"
},
{
"__attrId": "attr-12"
},
{
"__exprId": "expr-7"
}
]
}
},
{
"id": "op-1",
"name": "Project",
"childIds": [
"op-2"
],
"output": [
"attr-13"
],
"params": {
"projectList": [
{
"__attrId": "attr-13"
}
]
}
}
]
},
"attributes": [
{
"id": "attr-0",
"dataType": "e63adadc-648a-56a0-9424-3289858cf0bb",
"name": "id"
},
{
"id": "attr-1",
"dataType": "75fe27b9-9a00-5c7d-966f-33ba32333133",
"name": "content"
},
{
"id": "attr-2",
"dataType": "e63adadc-648a-56a0-9424-3289858cf0bb",
"name": "goods_id"
},
{
"id": "attr-3",
"dataType": "e63adadc-648a-56a0-9424-3289858cf0bb",
"name": "kdt_id"
},
{
"id": "attr-4",
"dataType": "e63adadc-648a-56a0-9424-3289858cf0bb",
"name": "score"
},
{
"id": "attr-5",
"dataType": "e63adadc-648a-56a0-9424-3289858cf0bb",
"name": "group_id"
},
{
"id": "attr-6",
"dataType": "e63adadc-648a-56a0-9424-3289858cf0bb",
"name": "score_level"
},
{
"id": "attr-7",
"dataType": "75fe27b9-9a00-5c7d-966f-33ba32333133",
"name": "created_at"
},
{
"id": "attr-8",
"dataType": "e63adadc-648a-56a0-9424-3289858cf0bb",
"name": "final_score"
},
{
"id": "attr-9",
"dataType": "e63adadc-648a-56a0-9424-3289858cf0bb",
"name": "comment_rerank_score"
},
{
"id": "attr-10",
"dataType": "75fe27b9-9a00-5c7d-966f-33ba32333133",
"name": "updated_at"
},
{
"id": "attr-11",
"dataType": "e63adadc-648a-56a0-9424-3289858cf0bb",
"name": "comment_origin_score"
},
{
"id": "attr-12",
"dataType": "75fe27b9-9a00-5c7d-966f-33ba32333133",
"name": "par"
},
{
"id": "attr-13",
"dataType": "75fe27b9-9a00-5c7d-966f-33ba32333133",
"childRefs": [
{
"__exprId": "expr-7"
}
],
"name": "comment_info"
}
],
"expressions": {
"functions": [
{
"id": "expr-2",
"dataType": "ab4da308-91fb-550a-a5e4-beddecff2a2b",
"childRefs": [
{
"__attrId": "attr-12"
}
],
"extra": {
"simpleClassName": "Cast",
"_typeHint": "expr.Generic"
},
"name": "cast",
"params": {
"timeZoneId": "Asia/Shanghai"
}
},
{
"id": "expr-1",
"dataType": "a155e715-56ab-59c4-a94b-ed1851a6984a",
"childRefs": [
{
"__exprId": "expr-2"
},
{
"__exprId": "expr-3"
}
],
"extra": {
"simpleClassName": "EqualTo",
"_typeHint": "expr.Binary",
"symbol": "="
},
"name": "equalto"
},
{
"id": "expr-5",
"dataType": "ba7ef708-332f-54fd-a671-c91d13ae6f8e",
"childRefs": [
{
"__exprId": "expr-6"
}
],
"extra": {
"simpleClassName": "Cast",
"_typeHint": "expr.Generic"
},
"name": "cast",
"params": {
"timeZoneId": "Asia/Shanghai"
}
},
{
"id": "expr-4",
"dataType": "a155e715-56ab-59c4-a94b-ed1851a6984a",
"childRefs": [
{
"__attrId": "attr-11"
},
{
"__exprId": "expr-5"
}
],
"extra": {
"simpleClassName": "GreaterThanOrEqual",
"_typeHint": "expr.Binary",
"symbol": ">="
},
"name": "greaterthanorequal"
},
{
"id": "expr-0",
"dataType": "a155e715-56ab-59c4-a94b-ed1851a6984a",
"childRefs": [
{
"__exprId": "expr-1"
},
{
"__exprId": "expr-4"
}
],
"extra": {
"simpleClassName": "And",
"_typeHint": "expr.Binary",
"symbol": "&&"
},
"name": "and"
},
{
"id": "expr-8",
"dataType": "75fe27b9-9a00-5c7d-966f-33ba32333133",
"childRefs": [
{
"__attrId": "attr-3"
},
{
"__attrId": "attr-0"
},
{
"__attrId": "attr-8"
}
],
"extra": {
"simpleClassName": "PythonUDF",
"_typeHint": "expr.Generic"
},
"name": "pythonudf",
"params": {
"name": "fun_one",
"evalType": 100,
"func": "PythonFunction(WrappedArray(...),{PYTHONPATH={{PWD}}/pyspark.zip<CPS>{{PWD}}/py4j-0.10.9-src.zip, PYTHONHASHSEED=0},[],/data/venv/hdp-envpy3/bin/python,3.6,[],PythonAccumulatorV2(id: 0, name: None, value: []))",
"udfDeterministic": true
}
},
{
"id": "expr-7",
"dataType": "75fe27b9-9a00-5c7d-966f-33ba32333133",
"childRefs": [
{
"__exprId": "expr-8"
}
],
"extra": {
"simpleClassName": "Alias",
"_typeHint": "expr.Alias"
},
"name": "comment_info",
"params": {
"name": "comment_info",
"nonInheritableMetadataKeys": [
"__dataset_id",
"__col_position"
],
"explicitMetadata": "{}"
}
}
],
"constants": [
{
"id": "expr-3",
"dataType": "455d9d5b-7620-529e-840b-897cee45e560",
"extra": {
"simpleClassName": "Literal",
"_typeHint": "expr.Literal"
},
"value": 20220830
},
{
"id": "expr-6",
"dataType": "455d9d5b-7620-529e-840b-897cee45e560",
"extra": {
"simpleClassName": "Literal",
"_typeHint": "expr.Literal"
},
"value": 80
}
]
},
"systemInfo": {
"name": "spark",
"version": "3.1.2-yz-1.4"
},
"agentInfo": {
"name": "spline",
"version": "1.0.0-SNAPSHOT+874577a"
},
"extraInfo": {
"appName": "team evaluation ranks",
"dataTypes": [
{
"_typeHint": "dt.Simple",
"id": "e63adadc-648a-56a0-9424-3289858cf0bb",
"name": "bigint",
"nullable": true
},
{
"_typeHint": "dt.Simple",
"id": "75fe27b9-9a00-5c7d-966f-33ba32333133",
"name": "string",
"nullable": true
},
{
"_typeHint": "dt.Simple",
"id": "a155e715-56ab-59c4-a94b-ed1851a6984a",
"name": "boolean",
"nullable": true
},
{
"_typeHint": "dt.Simple",
"id": "ab4da308-91fb-550a-a5e4-beddecff2a2b",
"name": "int",
"nullable": true
},
{
"_typeHint": "dt.Simple",
"id": "455d9d5b-7620-529e-840b-897cee45e560",
"name": "int",
"nullable": false
},
{
"_typeHint": "dt.Simple",
"id": "ba7ef708-332f-54fd-a671-c91d13ae6f8e",
"name": "bigint",
"nullable": false
}
]
}
}
]
[
"event",
{
"planId": "acd5157c-ddc5-5ef0-b1bc-06bb8dcda841",
"timestamp": 1661960765255,
"durationNs": 4353094937,
"extra": {
"appId": "application_1656468332243_128755",
"user": "app",
"readMetrics": {
"numFiles": 1,
"scanTime": 995,
"pruningTime": 0,
"metadataTime": 161,
"filesSize": 87538,
"numOutputRows": 7269,
"numPartitions": 1
},
"writeMetrics": {
"numFiles": 1,
"numOutputBytes": 48430,
"numOutputRows": 6785,
"numParts": 0
}
}
}
]
3.1.3 血缘分发
LineageDispatcher 决定血缘如何发送, 而内置的 Dispatcher 的实现也是一目了然的。
例如:HttpLineageDispatcher 就是将血缘发送给一个 HTTP 接口, KafkaLineageDispatcher 就是发给一个 Kafka topic, LoggingLineageDispatcher 就是将血缘打印在 Spark APP 的 stderr 日志里, 方便调试确认。
如果要自定义dispatcher, 可以自己继承 LineageDispatcher, 并且提供一个入参为 org.apache.commons.configuration.Configuration
的构造函数。
配置如下:
spline.lineageDispatcher=my-dispatcher
spline.lineageDispatcher.my-dispatcher.className=org.example.spline.MyDispatcherImpl
spline.lineageDispatcher.my-dispatcher.prop1=value1
spline.lineageDispatcher.my-dispatcher.prop2=value2
3.1.4 后置处理
post processing filter 可以在血缘解析完成后,交给dispatcher前进行一些后置处理,例如脱敏 。 实现一个 filter 需要实现 za.co.absa.spline.harvester.postprocessing.PostProcessingFilter
, 构造器接受 一个类型为 org.apache.commons.configuration.Configuration
的入参。
配置方式如下:
spline.postProcessingFilter=my-filter
spline.postProcessingFilter.my-filter.className=my.awesome.CustomFilter
spline.postProcessingFilter.my-filter.prop1=value1
spline.postProcessingFilter.my-filter.prop2=value2
3.2 版本关系
备注: 但是 pyspark 2.3 如果要支持 codeless init , 需要打个 patch SPARK-23228, 相关问题可以参考这个 ISSUE ( https://github.com/AbsaOSS/spline-spark-agent/issues/490 )。
3.3 spline 集成
3.3.1 集成 spline
编译 对应 spark 与 scala 版本的 spline-agent jar包。
如 spark 3.1
mvn scala-cross-build:change-version -Pscala-2.12
mvn clean install -Pscala-2.12,spark-3.1 -DskipTests=True
将 spark agent jar包部署在 /path/to/spark/jars 目录下。
配置 spark-defaults.conf
spark.sql.queryExecutionListeners=za.co.absa.spline.harvester.listener.SplineQueryExecutionListener
spark.spline.mode=BEST_EFFORT
spark.spline.lineageDispatcher=composite
spark.spline.lineageDispatcher.composite.dispatchers=logging,http
spark.spline.lineageDispatcher.http.producer.url=http://[ip:port]/producer
3.3.2 血缘收集系统
与现有系统集成要适当修改代码,在最后的 event 消息中添加该 Spark APP 对应的工作流或者任务名称, 将血缘和任务信息发给自定义的 HTTP server, 解析血缘上报 kafka, 统一消费处理。
3.4 spline 演示
通过docker-compose 可以一键启动 spline server 端。 可以通过 spline ui 看到解析出来的血缘。
1. wget https://raw.githubusercontent.com/AbsaOSS/spline-getting-started/main/docker/docker-compose.yml
2. wget https://raw.githubusercontent.com/AbsaOSS/spline-getting-started/main/docker/.env
3. export DOCKER_HOST_EXTERNAL=yourhostip // spline ui访问server默认地址127.0.0.1, 不修改无法外部访问
4. docker-compose up
四、总结
spline agent 能够无感知的为线上运行的 Spark APP 程序增加血缘解析, 是个很不错的思路, 可以基于这个方向进行进一步的研究优化。目前 spline agent 有一些无法处理的逻辑,如下所示:
1. 无法解析到 RDD 中的来源逻辑, 如果 dataframe 转换为 RDD 进行操作,则无法追踪到这之后的血缘。这跟 spline 解析的时候通过 logicalPlan 中的 child 关系进行递归有关, 遇到 LogicalRDD 递归结束。可能的解决方案是遇到 LogicalRDD 算子的时候通过解析 RDD的 dependency 关系查找血缘信息。
2. 血缘解析基于写入触发, 所以如果任务只做查询是解析不到血缘的
五、参考资料
- SPROV 2.0
- TiTian: http://web.cs.ucla.edu/~todd/research/vldb16.pdf
- Pebble
- Spline
- Atlas
- spline-spark-agent github
- Collecting and visualizing data lineage of Spark jobs
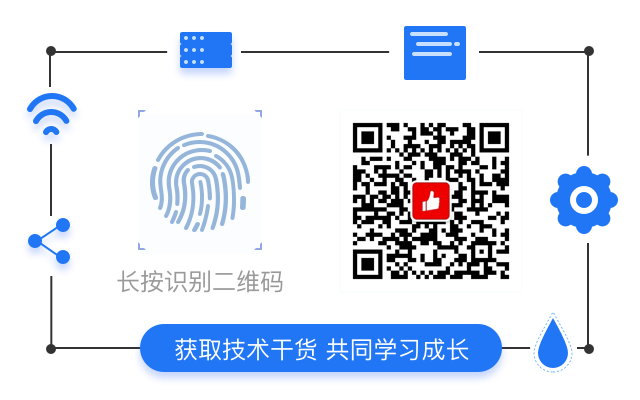